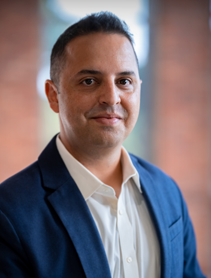
Dr. Brian Jalaian
- Position: Associate Professor
- Department: Computer Science; Intelligent Systems and Robotics
- Office Location: Campus: Building 4, Room 434;
- bjalaian@uwf.edu
- Campus: 850.474.2963
Biography
Dr. Brian Jalaian is an Associate Professor in the Department of Intelligent Systems and Robotics at the University of West Florida and a research scientist at the Institute for Human-Machine Cognition (IHMC). He obtained his Ph.D. and MS degrees in Electrical Engineering in 2016 and 2013, respectively, and an MS in Industrial and Systems Engineering (Operation Research) in 2014, all from Virginia Tech, Blacksburg, VA. He was formerly AI Test & Evaluation Tools Lead at DoD Joint Artificial Intelligence Center (JAIC), which is later merged into Chief Data Artificial Intelligence Office (CDAO). Prior to that, he was a research scientist at the Army Research Laboratory (ARL) leading research on the robustness and resiliency of AI. Dr. Jalaian has experience on forming and leading cross-functional machine learning teams to pursue research and development on cutting-edge technologies. His research experience, skills, and technical leadership impact the basic research on developing unique capabilities for artificial intelligence in Army Research Laboratory. Particularly, he formed and led a machine learning research team focusing on uncertainty quantification, safe, robust, and resilient artificial intelligence on the Internet of Battlefield Things Collaborative Research Alliance, which has been very successful and contributed to several knowledge products and highlighted the importance of risk-aware artificial intelligence at DoD.
His most recent research interests are robust machine learning, uncertainty quantification for machine learning, deep learning, AI safety and security, AI assurance, optimization, and network science. His research has been published widely in world class machine learning conferences such NeurIPS, ICML, AAAI as well as prestigious IEEE journals.
Degrees & Institutions
Ph.D. Electrical Engineering, Virginia Tech
M.S. Industrial Systems Engineering, Virginia Tech
M.S. Electrical Engineering, Communication & Network Systems, Virginia Tech
Research
- Large Language Models: Exploration of language models to enhance natural language understanding,
generation, and translation, with a focus on addressing bias, fairness, and explainability. - Neuro‐Symbolic AI: Integration of symbolic reasoning and deep learning approaches to enable more interpretable and explainable AI systems for complex decision‐making tasks.
- Bayesian Deep Learning and Uncertainty Quantification: Design and development of computationally tractable algorithms for Bayesian Neural Network approximation, with a focus on robustness, resilience, confidence calibration, and uncertainty‐awareness in AI systems.
- Large Foundational Models: Investigation and analysis of large‐scale foundational models to understand their capabilities, limitations, and ethical considerations.
- Adversarial Machine Learning: Development of defense mechanisms against adversarial attacks and exploration of robust AI models to enhance security and privacy.
- Domain Adaptation and Transfer Learning: Utilization of transfer learning techniques and domain adaptation strategies to improve AI performance in different domains and real‐world scenarios.
- Deep Learning for Anomaly Detection: Application of deep neural networks and unsupervised learning
techniques for detecting anomalies and abnormalities in complex datasets. - Semi‐Supervised Learning: Exploration of techniques that leverage unlabeled data to improve the performance and scalability of AI models with limited labeled data.